Uncertainty estimations don’t consider the unknown unknowns
There are two kinds of uncertainties. One of them are the uncertainties inherent to every measurement process. They happen because all measuring equipments are inherently flawed, including rulers, scales, Gamma-ray spectrometry systems, and human beings. And we can estimate how bad they’re flawed by testing them against primary standards in whose values we are pretty confident.
The problem is that the estimated uncertainty of a measurement can be severely underestimated because of the unknown unknowns.
“There are known unknowns, and there are unknown unknowns.” (Donald Rumsfeld)
When trying to infer the probability of an event going wrong, it is impossible to get a correct number because it is not possible to know, beforehand, all the unexpected sources of uncertainty—and they are infinite.
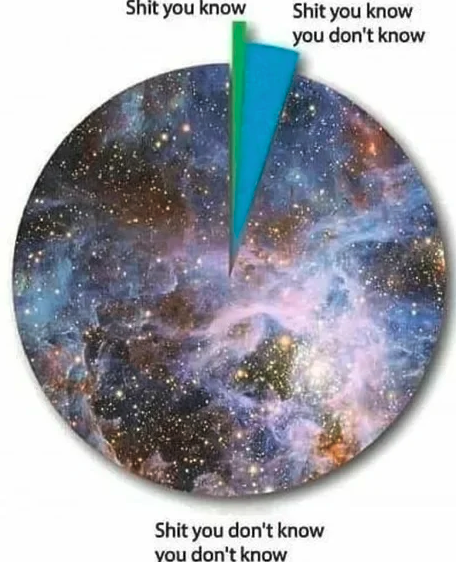
Suppose we have a production line that churns out an average of 1000 parts per day. Due to a number of variables, the factory produces sometimes more, sometimes less parts, but it’s always ± 4 for every 1000 parts with a 99,5% confidence interval.
Could you say that your factory is going to manufacture 30,000 ± 120 parts by the end of the month with 99,5% probability? Have you accounted for the chances of a key employee going nuts and throwing a wrench in the production line? Or your employees going on strike and lowering the factory’s throughput to zero? Or a pandemic?
According to David Chapman, no matter the area or context, people tend to react in the following ways when they face the unknown:
- They turn their backs to the unknown unknowns and hope for the best;
- They transform the real world so that it becomes less and less nebulous and more shielded against unexpected elements;
- They use common sense to keep their feet on the ground and assume that probabilistic inferences are fallible.
References
David Chapman (2019) The Spanish Inquisition
If you knew, before getting to the river, that the rowboat is in good working order, that the river is safe for boating, and so on, you could conclude that crossing would be possible. However, you cannot be certain until you get there and see. This defeats standard formal logic. (…) You might instead be able to reason probabilistically about “known unknowns”—obstacles that you could, realistically, anticipate and assign probabilities to. You could not, realistically, anticipate “unknown unknowns,” for instance that someone has filled your boat with electric eels, although it is logically possible that they did. This defeats probabilism.